Clinalytix: a New Approach to Risk Reduction
Key Topics, Use Cases, and More
Introducing two new use cases for the AI-driven data model
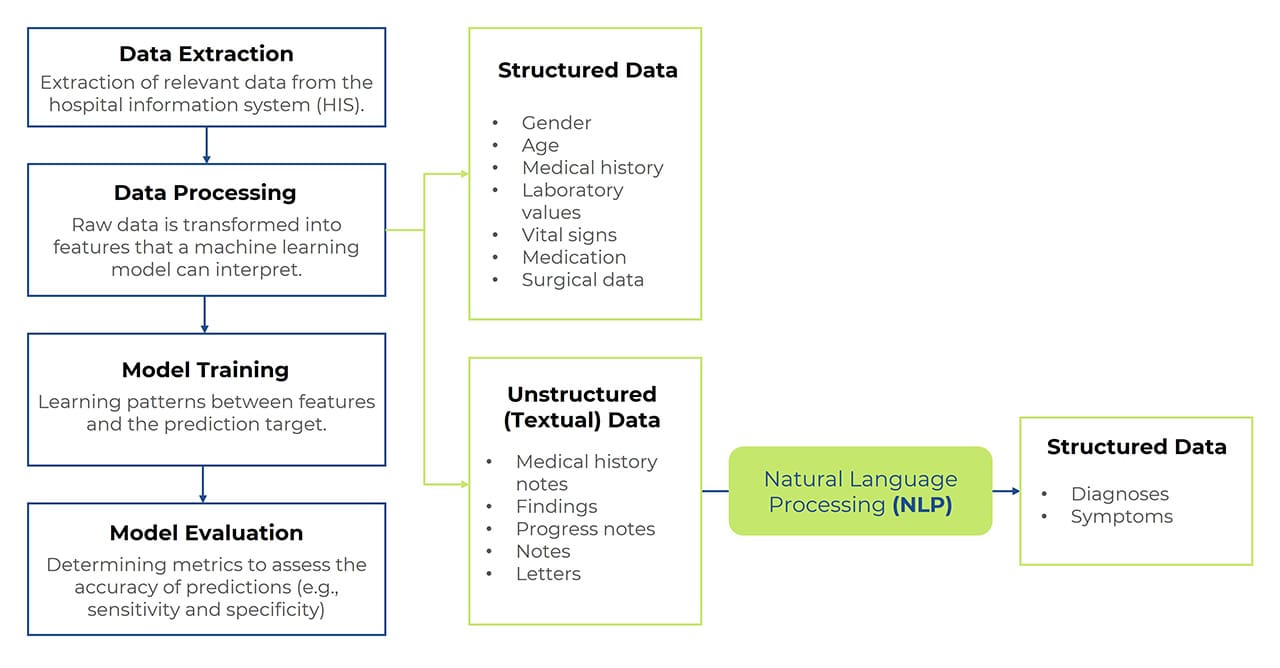
clinalytix focuses on risk prediction by identifying and mapping patterns within patient records. Its predictive model leverages neural networks to estimate the likelihood of disease development during a patient’s hospital stay. This prediction is based on available electronic health record data, represented through specific features, which may in turn be derived from structured or unstructured data. Extracting relevant information from unstructured data requires an additional processing step which uses natural language processing (NLP).
The system’s risk alerts, generated based on significant patterns in treatment data, enable early intervention and appropriate preventive measures, reducing complications and ultimately also hospital length of stay—leading to positive financial impacts.
The first certified medical device version of clinalytix covered two use cases: delirium and sepsis. Subsequent developments focused on significantly improving NLP capabilities and experimenting with various model variations. This foundational research paved the way for expanding the scope of the tool, and the latest version now includes two additional certified use cases.
New Use Cases: Venous Thromboembolism (VTE) and Pneumonia
Just like the existing use cases, these conditions are major contributors to hospital morbidity and mortality – and delayed treatment initiation, in particular, can have severe consequences for patients.
Two Additional Conditions: Deep Vein Thrombosis (DVT) and Pulmonary Embolism (PE)
Venous thromboembolism is a serious, yet often preventable, hospital-acquired complication which occurs when blood clots form in deep veins. The clots then potentially migrate and block other vessels, ultimately causing embolism. Given the limited mobility of many hospitalized patients, the risk of such complications is high, both during and after hospitalization.
On the other hand, unlike deep vein thrombosis, pulmonary embolism is life-threatening, with an estimated mortality rate of 28% if left untreated.
Pneumonia: Predicting Hospital-Acquired Cases
Pneumonia is a lung infection caused by bacteria, viruses, or fungi. clinalytix aims to predict hospital-acquired pneumonia (HAP) which developed at leas
The Importance of Early Intervention and Preventive Measures
Preventing Complications
- VTE: Thrombosis can lead to life-threatening pulmonary embolism, blocking the flow of blood to the lungs and causing severe respiratory distress, heart failure, or even death.
- Pneumonia: Delayed or untreated pneumonia can result in severe complications such as lung abscesses, pleural effusion, sepsis, or acute respiratory failure.
Reducing Length of Hospital Stay
Early and effective treatment of VTE and pneumonia accelerates recovery, allowing for earlier patient discharge. This reduces the strain on the healthcare system and lowers costs.
Lowering Hospital Mortality Rates
Both these conditions have high mortality rates, particularly among elderly and immunocompromised patients. Timely interventions therefore significantly improve survival rates.
Preventing Long-Term Health Issues
- VTE: Early treatment prevents thrombosis progression and reduces the risk of chronic venous insufficiency and post-thrombotic syndrome.
- Pneumonia: Rapid intervention halts infection progression, minimizing the risk of lasting lung damage.
Enhancing Quality of Life
Prompt and effective treatment alleviates symptoms faster, improving patients’ quality of life during and after hospitalization.
Impact of Early Treatment on Outcomes
Timely therapy for VTE and pneumonia plays a crucial role in improving clinical outcomes, reducing complications, and lowering mortality rates. clinalytix’s AI-powered risk prediction is automatically triggered during a patient’s hospital stay as new data becomes available in their electronic medical record.
Proven Effectiveness: ROC Curves and Model Accuracy
To evaluate effectiveness, independent test data is analyzed using receiver operating characteristic (ROC) curves. As more data becomes available throughout the patient’s hospital stay, the predictive model becomes increasingly accurate.
The area under the ROC curve (AUROC) is a widely used indicator of predictive model performance. At discharge, the AUROC value is higher, demonstrating improved accuracy. An AUROC score between 70% and 80% is considered indicative of a well-performing model, while scores above 80% reflect excellent predictive capability.
Model metrics suggest that high-quality predictions can be made shortly after admission.
These predictions serve as an additional tool for clinicians, supplementing established diagnostic methods rather than replacing them. The predictive service is seamlessly integrated into the ORBIS documentation and treatment workflow. Physicians can use the AI-generated risk assessment alongside traditional diagnostic approaches to make the best informed decisions about patient care.
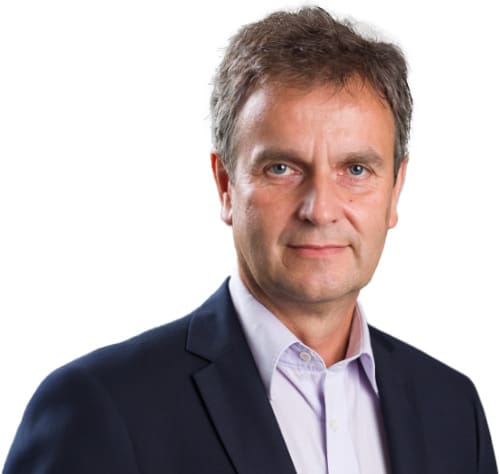
Ralph Szymanowsky
Business Development
TIP HCe