An innovative artificial intelligence-based method
to compress complex models into explainable, model-agnostic and reduced decision support systems with application to healthcare (NEAR)
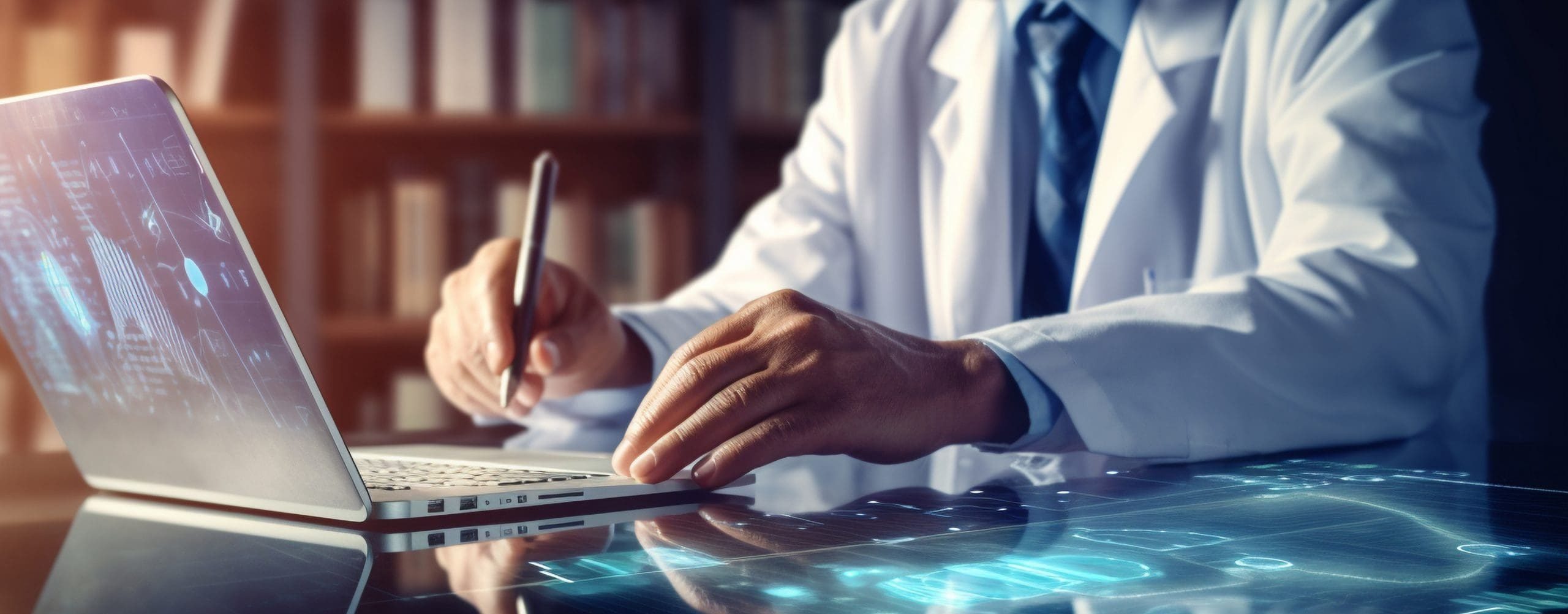
Transforming Healthcare Decision-Making: Introducing NEAR
Neural imputed Explainable and Adaptive Risk
In the fast-evolving landscape of healthcare, the need for personalized and precise medical decisions has never been more critical. Traditional clinical guidelines, while instrumental, are based on the characteristics of average populations and thus often fall short in catering to the diverse needs of real-world patients, in addition to being static and rigid by nature. In the era of precision medicine, this gap has highlighted the need for a paradigm shift towards individualized patient care. By making Clinical Decision Support Systems (CDSSs) more adaptable, explainable and accessible to healthcare professionals, this is the shift that NEAR – an innovative AI-based methodology – seeks to achieve.
The NEAR Approach: A Leap Towards Explainable AI in Healthcare
At the heart of NEAR is the Shapley Additive Explanations framework (SHAP), a sophisticated tool that dissects complex AI models to reveal precisely how, and to what extent, each input feature influences the overall decision-making process. This method not only demystifies the “black-box” nature of AI algorithms but also enhances the system’s transparency, making it more understandable, interpretable and trustworthy for clinical use. NEAR’s ability to compress AI-based prediction models into simpler, model-agnostic forms without compromising on accuracy is a testament to its revolutionary design.
Validating NEAR: A Real-World Application
NEAR was evaluated through an application to a real-world healthcare use case – the mortality prediction in patients who experience Acute Coronary Syndrome (ACS). By highlighting the comparable prediction performance of NEAR and of the sophisticated ML/DL models upon which NEAR was built (AdaBoost, FFNN, and customized NB classifier), this assessment validates the efficacy, the relevance and the precision of NEAR in a real, critical, clinical use case.
Explainability and Accessibility: The Cornerstones of NEAR
By offering a CDSS that is both tailored to single-patient analysis and inherently modular, NEAR paves the way for more dynamic and personalized clinical guidelines, thereby assisting doctors in making informed decisions in their day-to-day practice. NEAR’s significant asset is its explainability, while additionally providing clinicians with information as to what constitutes the final risk and its composition – which makes it all the more relevant for clinical decision-making. Lastly, NEAR can be accessed via an intuitive, user-friendly web-based platform, which makes it even more explainable, transparent and ultimately, invaluable for healthcare professionals.
Conclusion: A Future with Personalized Healthcare Decisions
The development of NEAR represents a major step towards integrating AI-based systems within the healthcare sector, addressing the critical need for explainable and reliable CDSSs and augmenting patient care. By offering a solution that is both innovative and practical, NEAR has the potential to revolutionize how medical decisions are made – enhancing practices, aligning with the highest standards of care and with the principles of precision medicine, all while ensuring that care is tailored to the unique circumstances of each patient. Thanks to advancements like NEAR, the future of healthcare, more personalized, dynamic and informed, is really just around the corner.
Keywords: Clinical Decision Support Systems, Explainability, Artificial Intelligence, Precision Medicine, Risk Prediction